On-demand webinar
What is “Artificial Intelligence” in Customer Service?
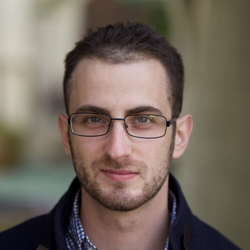
Maksim Gill
VP Product, Eccentex
Discussion Topics:
- The gray area between AI, business rules and automation
- Real examples of how AI supports customer journeys
- Breaking siloes is the key to a differentiated, personalized AI service
- How to keep AI-assisted conversations feeling human
- Building the ideal AI-assisted agent desktop
Most companies are already employing some level of automation and intelligence within their customer service organization. But getting to that next level of customer experience requires breaking down data and organization siloes.
Join Maksim Gill, VP of Product at Eccentex, demonstrate the ideal next step in deploying AI for customer service.
View Transcript
Max Gill (00:03):
My name is Max Gill. I’m the head of product at Eccentex. And I’ll be talking about journey-centric AI today. We can turn your attention to the picture on the right before we get started. My background is primarily in case management and the local platform space. And I run Eccentex app-based product team, which is our platform for delivering what we call digital business apps. Our customers use our platform to build applications like customer service, help desk, complaints, claims management, back-office automation, and much more. Because we’re oftentimes the glue between the front office, the back office, and the department’s intermediate, we’re able to track what the customer wants to do throughout its entire journey. And we’re going to see in this webinar today, how being able to track the entire journey provides a unique opportunity to use AI in assisting the employees. Or we call that merging the human workforce with the digital workforce.
Max Gill (01:15):
We work with companies and government agencies worldwide to help not only deliver these types of applications but to enable them to continuously improve them or make new apps. Before I actually start talking about artificial intelligence, I want to quickly talk about automation in general because oftentimes the goal of an AI project in an organization is to gain some sort of automation. An example of non-AI-driven automation would be something like the system emailing the customer after the complaint order automatically. This has some sort of AI in it, which is someone trying to deposit a check through a banking app where the system will automatically make sure that the images aren’t blurry, it can even extract what was written handwritten on these checks and compare it to what the person wrote in the app to make sure the check is real. There’s also a very complex use case of actually having a foreign conversation with a customer through a chatbot, for example.
Max Gill (02:22):
That is also automation because it’s automating what would have been a conversation between a live agent and a person. And those chatbots you’ve probably seen today can understand natural language. They can help guide you through a process. They can help show you where things are and initiate a refund, really do a lot of things. And on the slide, we see the customer-centric view of an organization, and there’s a lot of processes that the customer can be involved in, sometimes during multiple processes at once. And there’re lots of opportunities within each one of these processes to automate whether that automation is AI or not AI is a whole different question. We’re going to actually tackle that throughout this presentation. We’re also going to talk about employee-assisted AI because while there’ку lots of opportunities for automation, of course, in general, there is a lot of opportunities for artificially intelligent assistance to an employee. For example, non-AI assistance would be automatically loading everything about the customer onto the agent’s desktop screen, when the customer calls the call center. That’s a form of non-AI driven automates what would have been a need for the agent to look up the person’s name, to click between different systems. More AI things like suggesting the next best actions for a specific problem while speaking to the customer, which is more AI-driven.
Max Gill (04:11):
Throughout this webinar, we’re going to explore where there’re opportunities for automation within business rules versus artificial intelligence and how those two can work together. So we’re going to start with a typical journey of the customer can be on in this situation. A farmer is interested in purchasing a tractor but also needs to get financing either from the bank of an attractive company to do so. And we call it a journey, not a task or a process, because it’s more complicated than just that. It’s a whole journey. And the journey begins actually not when they apply for the loan, but it begins when they’re actually just interested in purchasing the tractor because that’s the first opportunity that the bank or the tractor company has to start marketing to the farmer. Assuming that the farmer is tech-savvy, finds a proper bank, goes to the bank’s website, fills out the form, maybe has a couple of chats here or there. They fill everything out. Everything is done. They submit.
New Speaker (05:14):
And because they are very well qualified for the swollen and because the bank has all the proper integrations and things like that, it’s a group prequalification. The farmer then gets a bunch of paperwork. They fill it out online and the money gets transferred to their account. This seems like a barely straightforward process. There’re clearly opportunities for automating most of it in this linear fashion, of course. Maybe there are some exceptions here or there, but it looks simple enough that we can model it out and we can use AI or non-AI tech if we should do it. The problem is that it doesn’t really go that way. In reality, the journey looks close to this.
Max Gill (06:05):
We can see that the complexity here is much higher than originally seen from just a high-level view. There’re a lot of touchpoints between the farmer and the bank. They can start a chat and end in a call. They may chat multiple times or call multiple times throughout the day. They may email. They may even use more modern communication channels like Facebook messenger or Twitter. They may start the application multiple times and the band in it before they actually end up completing it. And when they do complete it, if the score is not phenomenal or terrible to automatically disqualify, there is additional work that needs to be done to actually decide to give the one or not. People have to get involved, more cases have multiple tasks with multiple stakeholders that need to verify or approval like this.
Max Gill (06:57):
The bank may actually outsource parts of these tasks to different vendors or countries even. Then oftentimes a bank requires that things be signed physically. So they may mail documents to them, and then you’re required to come into the bank. Things get notarized. They may send a form out to get signed, but the farmer forgot to sign one part of it, they send it back. Then the bank has to contact them again. They may request additional verifications like proof of insurance. They may want bills of sale to make sure that they have a viable way of paying back. We can see it’s a pretty complex process when all the opportunities and exceptions are taken into account and it gets even more complicated if the farmer is on multiple journeys at once with the same bank. For example, they may be on a dispute journey with them because they have a credit card with the bank and they are trying to dispute a charge while at the same time trying to get a new loan.
New Speaker (07:53):
So even from a conversational AI standpoint, when the farmer calls in, it may not be clear what they’re calling about and that complicates the way that the conversational AI can respond adequately. And if the farmer is frustrated because they’re calling back multiple times and they can’t get the answers they want, having an AI to take care of that entire engagement part of it is high risk because one bad customer service experience is a lot more detrimental than a number of good ones. But AI is really good at a number of parts here that help automate this process, or at least assistant automating it. So from a little hanger or a more obvious way, AI can help in scoring. Obviously, there’re score mechanisms are already in place. Some of them are more structured, rules-based while others have machine learning in them that can analyze the behavior of how farmers and their demographic are actually paid back, maybe, they use more big data capabilities like a forecast that produce prices.
New Speaker (08:59):
All something that a human can theoretically do, but with so much data coming in and so much variety of data coming in, it’s just very difficult for a human to sit there and model it. AI is great for enhancing that type of scoring mechanism. We also see a lot of opportunity for when a call is made or a communication channel was made between the farmer and the bank in prioritizing that case or that conversation. If, for example, during the automated portion of a call, the voice structure of the farmer is seen to be emotional or angry that may place them at a higher position in the queue to actually talk to a real person. Same thing with emails. If there’s a nasty written email about how frustrated they are, that may go higher in the queue for some way to look into. And we see AI’s huge role in assigning work that would call predictive routing, which is assigning work to the correct agent to speak to or down the line to the correct people in the back office to process work.
Max Gill (10:05):
So we can use either the simple things with no AI, like just who the least busy person is, or we actually can use AI to understand what type of person may be their skillsets. Maybe some people are better at handling more angry people or are better at handling people in a specific region or a type of equipment that they’re trying to finance. Maybe that they have special knowledge about that. AI can really go through a lot of behavior and kind of track how every employee handles different tasks to determine what type of tasks are best for them to complete. There’re, obviously, opportunities for non-AI-driven automation. For example, when you actually do end up calling or speaking to an agent, pre-loading all the information about the customer on the agent’s screen is a great way of automation, not usually AI-driven generating communications back and forth, such as generating the summary of a call or automatically sending or resending a verification form out and to what we would like to call employee assisted AI, which is a great opportunity for AI in general. It doesn’t do a specific task so much, it just gives additional information or additional insights and employees while they’re working on a case like this.
Max Gill (11:24):
For example, if the customer isn’t a number of journeys, and they do call an agent, the system, the AI may predict what the person is calling about and thus preloading all the information or giving insight about what the situation is to the agent before they even pick up the call. Or while they’re actually during the call or in the back office working on this case, providing the next best actions where the AI can investigate how the previous cases were resolved, how successful they were, how they were not successful maybe. The system can say that in most situations they can exclude this type of verification because when they get them completely later on thus saving time. So next best action is a huge opportunity for AI here as well. And maybe even something slightly less AI-driven, but still has some AI is the recommendation of knowledge-based articles that the employee can read while on the call or while working on the case.
Max Gill (12:23):
For example, you can see, it automatically takes things like the region, the type of equipment, the state that the case is in right now to provide the most up-to-date information that can be read by the employee. So we’re going to be using customer service as an example because it’s a great way to illustrate employee-assisted AI. Up here I have a quote from Microsoft. It says “By 2025, as many as 95 percent of all customer interactions would be through channels supported by artificial intelligence (AI) technology”. And they don’t mean that all interactions would be through AI, but that it may be employee-assisted AI or completely AI.
Speaker 3 (13:09):
As we saw in the previous slide, that there are many interactions that still occur and will continue to occur where AI alone is not applicable. For example, those complex situations, those situations with high emotions or high risk for the company. On average in the industry, it costs about a dollar a minute to talk to somebody on the phone. So just remember that next time you have a problem with one of your cell phone providers, and you spent 43 minutes trying to argue over a $10 surcharge you don’t agree with. But even other channels like chat. Chat costs about half of that per minute because the Asian could do multiple chats at once.
Speaker 3 (13:51):
Let’s do it 50 cents a minute. It’s still pretty expensive. And self-service that’s considered to be 10 cents or cheaper essentially per task or per action you try to do. That includes both just going on the portal and filling out some stuff or actually having a conversation with a chatbot. Clearly, there is a push from actually talking to an agent on the phone to self-service, but it’s not always possible. So what we can do here is with employee-assisted AI we can provide additional insights or additional information to make the call faster, provide a better level of service, or just, in general, giving more information to the employees that they can provide a level of care that the person or the customer feels is more personalized to them.
New Speaker (14:40):
With advances in a natural process, language processing we also have the opportunity for the robots to listen in a real conversation and provide the right suggestions to the employee during the actual conversation. I also want to note that a conversational AI is not always applicable even for simple situations in cases where there is a high empathy need or an actual emergency risk. For example, if you’re calling an insurance company because of a death, even if the process is pretty straightforward, the emotional level of the customer is very high and you can’t recreate much empathy between a robot and a human these days. And sometimes in an emergency, for example, 911 or you have a gas leak or something that is also something that people genuinely want to speak to a human with.
Speaker 3 (15:32):
And this is one of my favorite jokes. I don’t particularly agree with all of it, but I think it’s pretty fun.
Max Gill (15:41):
We’re going to dive into an example right now. We’re going to quickly step away from the farmer journey and just talk about a general customer support journey that a customer may be on with a bank. So in this situation, somebody calls a bank to complain about a fee that they don’t agree with. From an AI standpoint, obviously, as you’re talking to the robots, you can do analysis right away, understand how angry they are, maybe also analyze the customer value from the bank standpoint and prioritize correctly within the call queue. But then when actually guess the agent to actually assist the agent with data and insights that would help them not only solve this person’s problem but also solve their additional questions they may have, which is what we would consider being a super agent. And everybody expects that these days, that when you call somebody, you don’t get transferred from the department to department, this one person should be able to somehow solve all of your problems.
Max Gill (16:41):
There’s also a big push in the industry to make customer service also a profit center or revenue-generating center. You may end up calling the bank to raise your credit limit on a credit card, and they may end up actually selling you another credit card. Or if you’ve ever traveled and you called to inquire about their national call rates, but actually ended up signing up for a plan that you weren’t even expecting to do. Having agents that are capable of solving many of the customer’s problems without transferring them and upselling them is a pretty high bar to reach. They may not have all the training to do it. They definitely don’t have the time to review all the journeys and all information about the customer for the call comes in. So AI-assisted employees is a great way to enable the super agents. This is a screen that an agent may see when a customer actually calls in. It’s a lot of information. I pause here for a few seconds.
Max Gill (17:51):
So the agent sees pretty much all that information they need in order to answer any questions the customer may have and do additional actions if necessary. This mashup of all this information, all these actions we call it the customer 360 view. And this in itself is a form of automation, we had situations where we are trying to tell them, tell an agent about something. And they’re saying, Oh, wait a minute, I have to go to look in this system, I have to go in that system. All this clicking between systems and that itself is manual work, that’s prone to error and takes time. Automating the collection of all this information to one screen is critical to providing a good customer experience. For example, here we have a list of all the transactions that the customer has had. Maybe any products they’d had, any ongoing things can be here as well, actions that the customer has been calling about or needs.
Max Gill (18:52):
Unless you know specifically what they’re calling about, you may be having a complaint and the need for a credit line increases. There are multiple things that are happening. So just being able to quickly create everything from one place and stuff that goes in the back office or other departments all in one place is also a form of automation. If we zoom out a bit, we also see a very important part here right in the middle which explains all the other journeys the customer has been on. And this is perhaps the most important, not only for the agent’s sake but for any AI that happens along the way. Here we see that the customer has previously called about a complaint about fees before, maybe another account complaint to try to open an auto loan, but then that got denied because the credit score was too low, tried it before once, but actually abandoned it.
New Speaker (19:47):
We have some insight into what the customer’s behavior is at this bank in general. We also see the results of all of these, and how long different steps take. And this is also important to kind of understand where the customer’s calling coming from and what he potentially would be calling about. From an AI standpoint, did he have any sort of opportunities to emotional analysis done while they’re talking with the robot before they actually got connected to the agent? You can always put that information up here in the summary section, maybe provide insight, Oh, this person is frustrated or this was a third time calling within the last couple of days. If there’s any indication of what they need to be calling about, it can also go here and already give the agent some sense of what’s going on during this call.
Max Gill (20:40):
For a second I’m going to focus on one part of the screen here primarily, which is going to be the suggestions portion. This is sometimes called next best action, sometimes suggestions. Without knowing right away what the customer’s calling about these are just things that help guide the agent or may offer opportunities to upsell or do preventive maintenance on the account. The first thing that we see is the fee protection service. This was offered because this customer accesses a few complaints twice already in this year. The fee protection service really only makes sense if it is actually the customer’s fault and not the bank’s fault. So we had two complaints here. One time it was no action, so the customer had to pay. And the second time there was a fee reduction after investigation.
Max Gill (21:34):
This type of fee protection service only makes sense if the customer perceives that it’s their fault and that it’s going to happen again. Even if in reality both times it was the customer’s fault, but for account management and for just keeping a happy customer they may have reduced the fee and made it seem like it’s their own fault and not their fault. Those types of things will affect whether or not this offer even makes sense, because again if you offer them a protection service for something that they perceive is your fault anyway, they’re not going to take that very well. We also can use additional information for the second offer here auto loan. Their credit score has increased based on some of the information that the bank has access to. We’ve seen that the person has requested a loan before one time they abandoned it.
Max Gill (22:30):
Other times they actually got denied because of a low score. Perhaps, based on analytics in general, knowing that customers of this type of demographic are maybe willing to buy a car, maybe they know their car is getting old, maybe they know other information about that demographic that would make this up a potential offer that is important enough to mention to the customer. We zoom out here for a second. We’ll see many opportunities for AI in general on the screen. You can’t just provide an unlimited number of offers to the customer. Not only because it takes a lot of time because it will frustrate the customer and they’d be calling about a problem and they may get unhappy that you’re also trying to sell them something. If you should only really focus on upselling that is going to be most likely a hit.
Max Gill (23:24):
Having AI be able to go through all the journeys and to understand customer’s feelings in general towards various offers, use AI to then have different data from different customers at different demographics, also the inputs to this is a driving force behind upselling for customer service. Of course, you can make bow it down to just being a business rule. For example, if you see that a customer has had two excessive complaints this year, and both times they resulted in them thinking that it was their fault, that automatically makes them suffer, but you can see that the number of different correlations that can occur like that, or the number of exceptions, the number of business rules, for example, excessive complaints, or maybe they have three excessive complaints and the two of them were his fault.
Max Gill (24:19):
At what point does it become clear enough for you to actually write it down as a business rule? You have all of these business rules are too hard, and AI is great at these types of analysis because it can analyze millions and millions of customer transactions. As you’re measuring, offering these things that you can offer a free protection service. And if they deny it, you can also put that in the system. The system knows that you’ve tried it and it then works. And it can use that for future predictions as well, not only for this specific customer but for future customers. It’s important to know that the suggestions can change throughout the call. Or you can imagine that these are the suggestions that are offered at the start of the call based on the AI’s interpretation from before.
Max Gill (25:10):
If I end up actually creating a fraud case during this call, those suggestions must change because it doesn’t make sense for you to start offering a new auto loan, if you’re just complaining to the bank about someone stealing your money. And simply writing out all of those business rules. If these suggestions exist, but then this happens then remove that suggestion or add a new suggestion. Those types of business rules are vastly limited. You may be able to do a lot of them as business rules, actually write them out, have matrices and business rules engines that do that. But the number of variations that can occur and also adding in the additional information like emotional analysis, text analysis makes it very difficult to constantly update those business rules.
New Speaker (26:03):
This is where AI is a huge help because it can continuously learn about what kind of offers were made throughout every call, what type of offers worked in the ward by the agents. And just in general be able to interpret the type of data that a human doesn’t have time or the ability to manage. There’s a huge benefit to being able to structure what was done during a call, during their processing of a case or the entire journey. Simply knowing that an offer was being offered to a customer during a specific type of journey can really help the system in the future know that next time in this type of journey these offers should be adjusted. One of the biggest necessities in order to have a system like this is being able to track the journeys from end to end, and being able to correlate actions that the agent or customer has taken against those journeys. And with that thank you for joining.
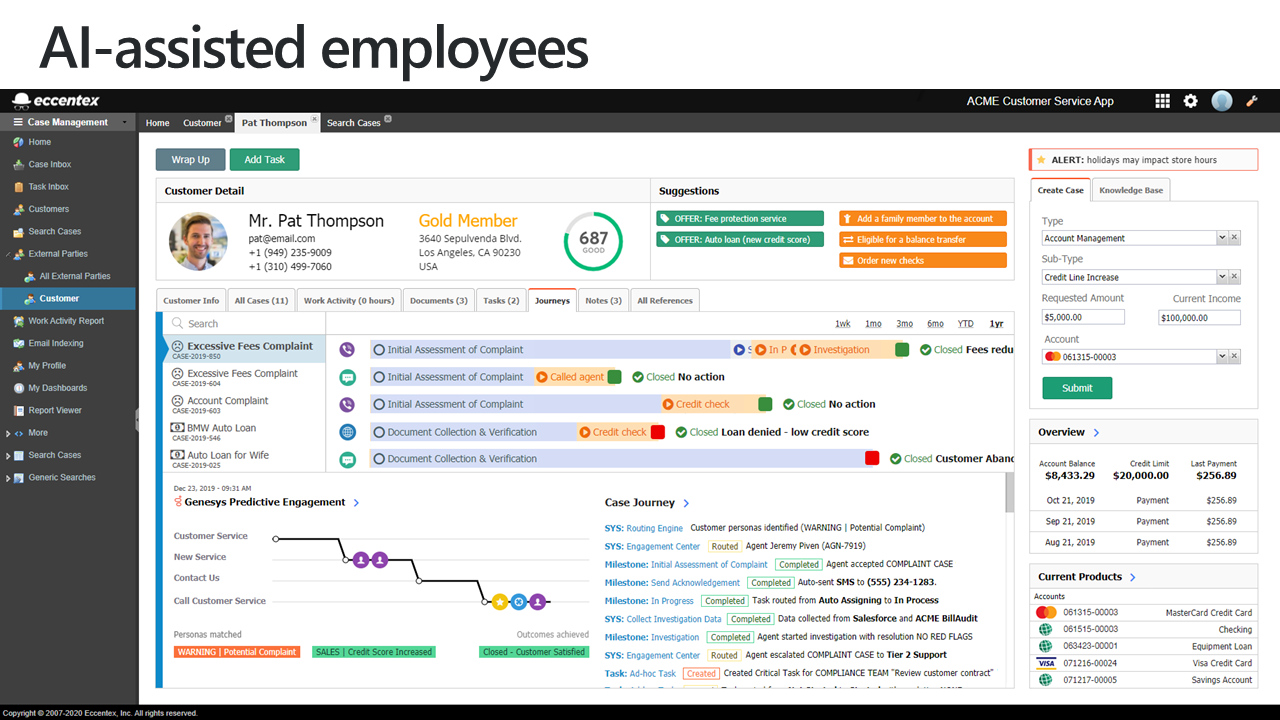
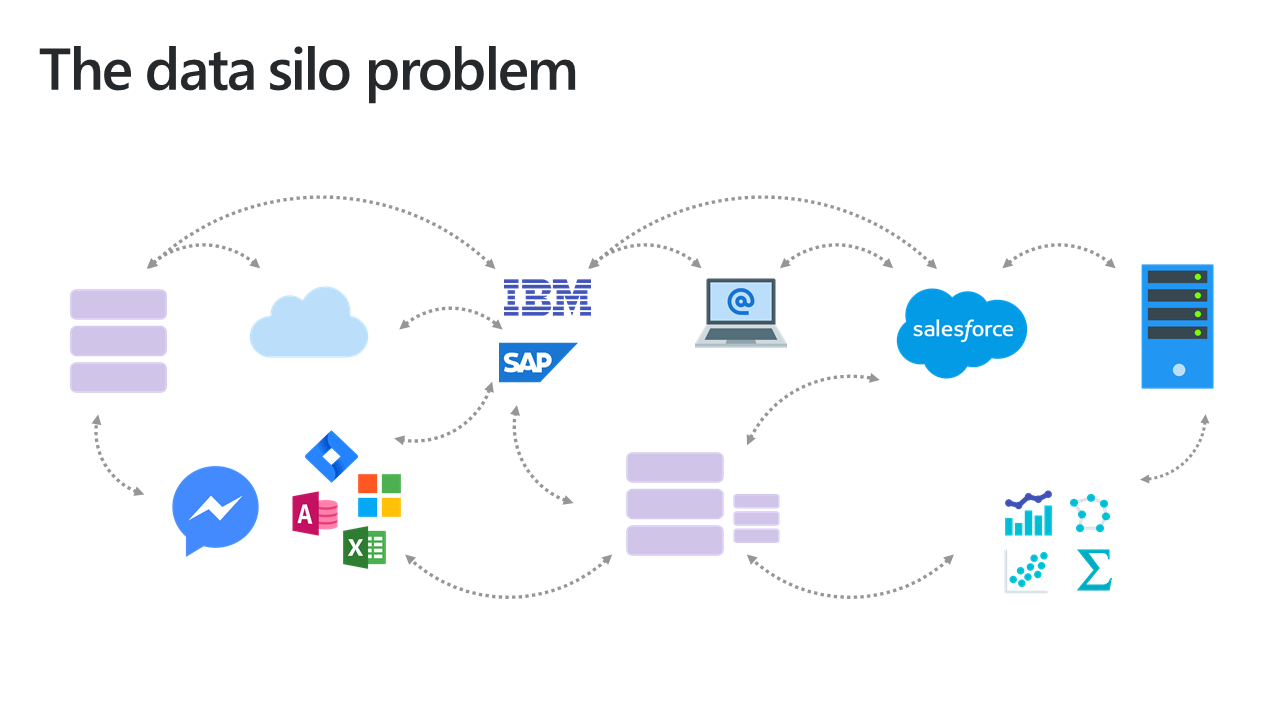
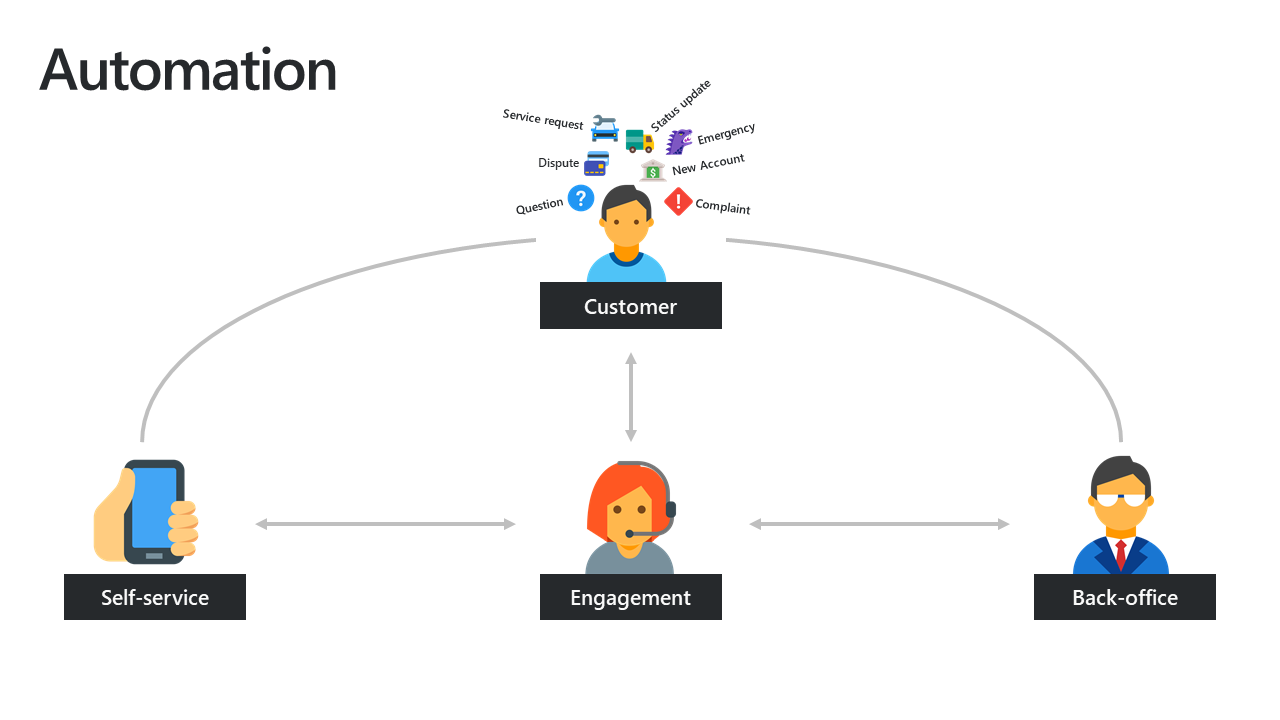
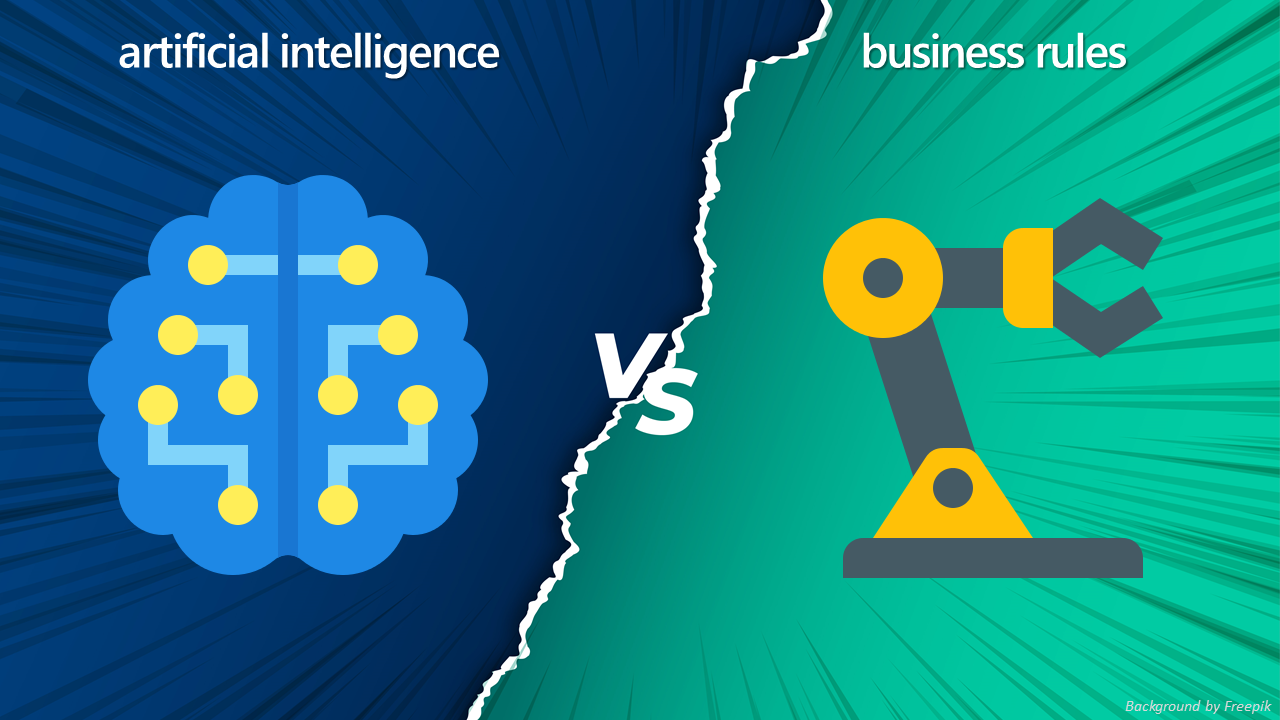
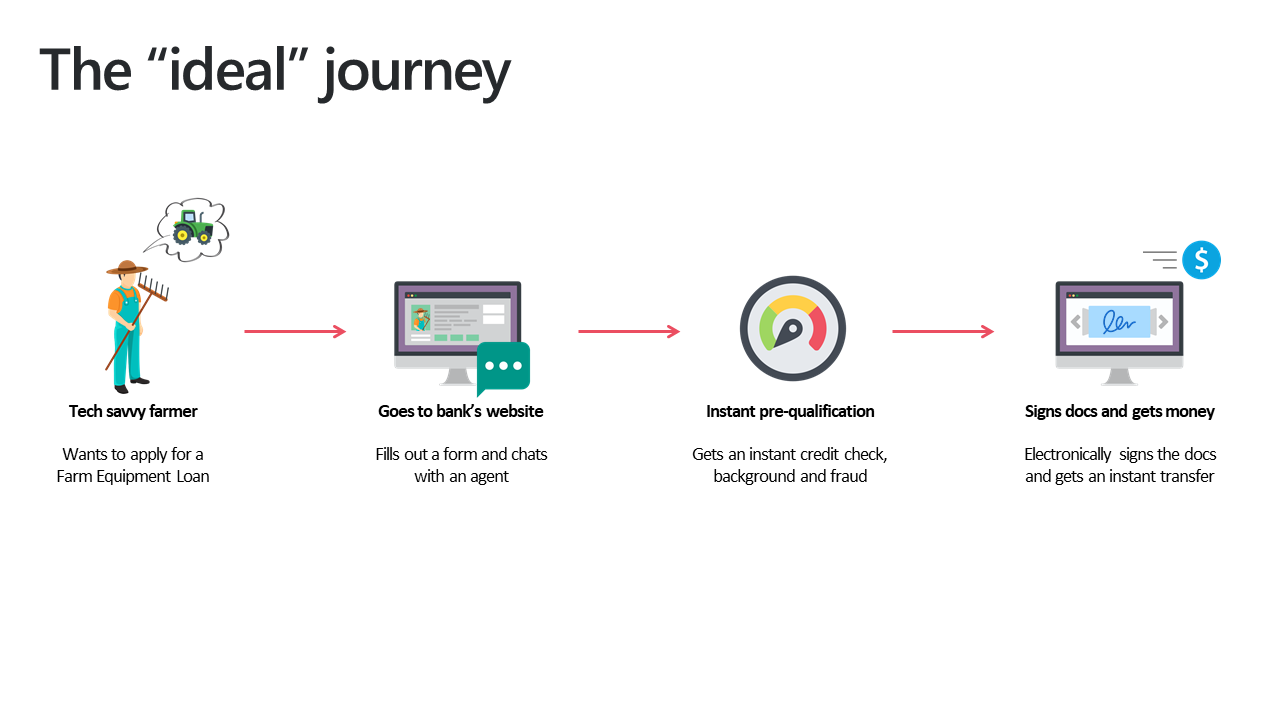
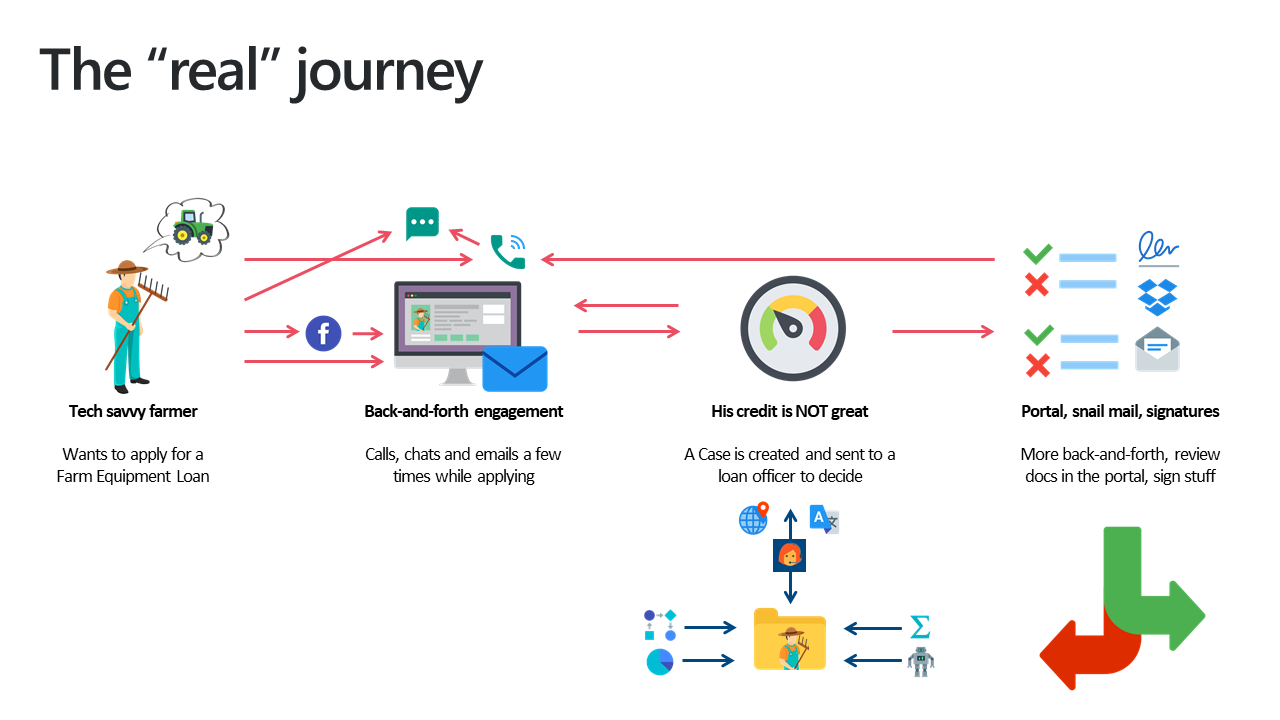